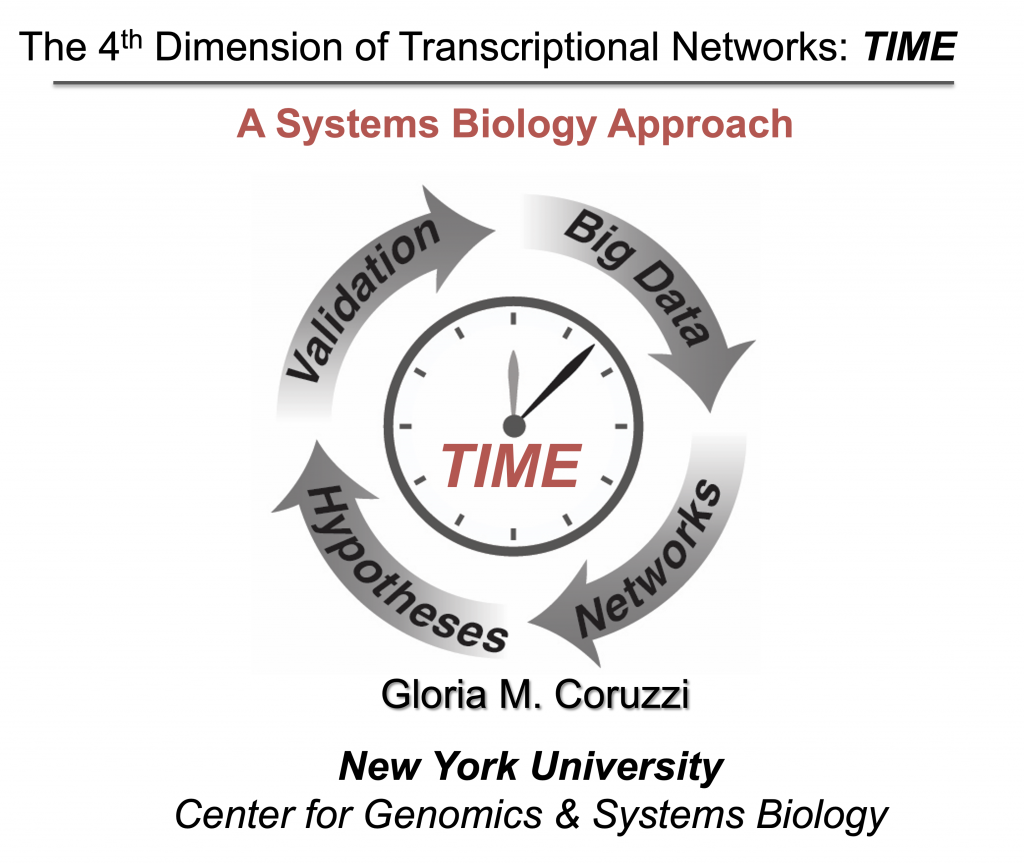
The 4th Dimension of Gene Regulatory Networks: TIME
Rapid reprogramming of transcriptional networks enables cells to adapt to a changing environment [1]. However, most gene regulatory networks (GRNs) are captured and modeled as a static web of interactions. To fill this gap, our studies exploit TIME – the relatively unexplored dimension of gene regulatory networks (GRNs) to uncover the temporal transcriptional logic that underlies dynamic nitrogen (N) signaling in plants [2-5]. Because causality moves forward in time, RNA-seq data from fine-scale time-series experiments are a valuable resource to infer GRN models that can predict TFtarget relationships in out-of-sample data – the ultimate goal in systems biology. To this end, we generated fine-scale time-series RNA-seq datasets from N-treated plants and used cubic-spline models to identify NxTIME response genes in shoots and roots of Arabidopsis [2]. Using these NxTIME gene sets, we developed a “just-in-time” approach that enabled us to uncover a temporal cascade of cis-elements underlying dynamic N-signaling [2]. Next, our goal was to identify the transcription factors (TFs) associated with this temporal cis-element cascade. To this end, we inferred TFtarget relationships from the NxTIME dataset using a time-based machine learning method – Dynamic Factor Graph (DFG) – which can infer gene responses at left-out time points [2,3]. To validate our time-based GRN models, we experimentally identified the genome-wide targets of 33 early N-response TFs using TARGET, a plant cell-based system we developed for temporal TF perturbation at scale [3]. These validated TFs include 9 TFs previously associated with in the N-responses in plants, as well as 24 novel TFs which TARGET assays validated to collectively regulate 88% of NxTime responsive genes in shoots and roots [3]. Importantly, we showed that TARGET can capture early and transient TFtarget interactions within minutes of controlled TF nuclear entry for NLP7 – a master TF in the N-response cascade [5]. We then used these validated TFtarget edges for 33TFs in two ways: 1) to develop a “Network Walking” approach which connects TF1TF2in planta (indirect) targets of TF1 [3,4], and 2) in a Precision/Recall (AUPR) analysis to identify high confidence TFtarget edges in our inferred GRNs [2,3]. These high-confidence GRNs now provide the temporal transcriptional logic for ~150 TFs and their targets in the dynamic N-signaling response in Arabidopsis [2,3]. In planta validation studies include master TFs in this GRN, where TGA1 regulates N-dose responsive plant growth via Michaelis-Menten kinetics [5], and NLP7 mediates its downstream in planta responses via early and transient target interactions in the GRN [4]. To broadly enable these approaches in the plant community, we created a platform called ConnecTF (https://connectf.org) that enables users to integrate many different datasets for TFtarget interactions (ChIP, Dap-Seq, RNA-seq) using TF-target data assembled from either Arabidopsis, maize or rice [6]. Automated functions in ConnecTF enables users using TF-target datasets to perform analysis for including “Network Walking” or to assess the accuracy of any inferred GRN using an automated AUPR analysis [6]. More broadly, these time-based approaches we have developed can be applied to uncover the temporal transcriptional logic for any signaling response system in biology, agriculture or medicine.
References
[1] Alvarez et al (2021) Annual Review of Plant Biology. Vol. 72:105-131.
[2] Alvarez et al (2020) Nature Communications. 11 (1), Article1157.
[3] J Swift et al (2020) Proc. Natl. Acad. Sci, USA. 117 (23) 12531-12540.
[4] Brooks et al (2021) Plant Physiology, Volume 185, Issue 1, January 2021, Pages 49–66.
[5]Swift & Coruzzi (2016) BBA Gene Regul Mech 1860:75,
[6] Li et al (2015) TIGS 31(9):509,
[7] Varala et al (2018) PNAS 115:6494,
[8] Greenham & McClung (2018) PNAS 115, 6325,
[9] Brooks et al (2019) Nature Comm. 10 (1) 1569,
[10] Krouk et al (2013) Genome Biology 14:123,
[11] Bargmann et al (2013) Mol Plant 6(3):978-80,
[12] Para et al (2015) PNAS 111:10371,
[13] Doidy et al (2016) BMC Genomics 17:92